Shoumik Saha
[CV]. [Google Scholar]. [LinkedIn].
Live and let live!
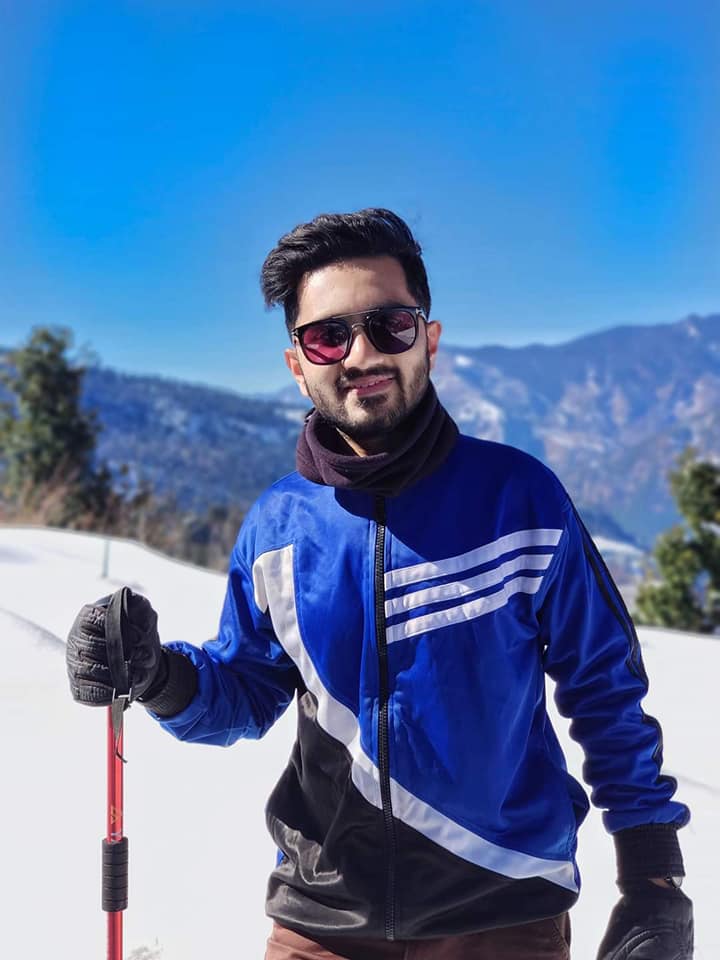
CS Ph.D. Student
UMD College Park
smksaha@umd.edu
I am a 3rd year Computer Science Ph.D. student at the University of Maryland - College Park, where I am fortunate to be advised by Prof. Soheil Feizi. Last summer, I have worked as a Scientist Intern at Amazon AWS. My research journey began with a focus on machine learning for security, particularly in malware detection. Over time, my interests have evolved toward security and reliability in machine learning. These days, I’m dedicated to enhancing the robustness and reliability of generative AI, especially large language models (LLMs).
If you check out my CV, you’ll see a consistent theme: I enjoy exploring challenges from both sides of the coin—attack and defense, red team and blue team, or however you’d like to frame it. Sounds interesting? Feel free to reach out to discuss my research or potential collaborations!
Before joining the Ph.D. program, I earned my B.Sc. from Bangladesh University of Engineering and Technology (BUET). I then gained valuable experience as a full-time lecturer at United International University and a part-time research assistant at BUET’s research lab.
news
May 16, 2025 | Research got featured in The New York Times! |
---|---|
May 15, 2025 | Paper on AI-text Detection accepted to ACL 2025! |
Jan 19, 2025 | Paper (co-authored) accepted to SaTML 2025! |
Dec 20, 2024 | Fall 2024: Completed all the coursework of Ph.D. |